Why does the world speak English? The awkward answer is, that over the years, Britain invaded most of it. While the country’s dominance as a world power may have dramatically receded, its linguistic legacy remains. This poses problems for technologies like AI that rely on English as the dominant mode of communication.
For AI to be truly effective, it needs to fully embrace the myriad of global languages. In multilingual countries like India, for instance, the ability of AI to understand and process varied local languages is key. However, achieving this requires more than just advanced technology—extensive data annotation needs to be in place for effective AI training. In this article, we’re going to look at the challenges this brings and explore solutions that will have AI speaking your language, no matter where you are.
The current challenges
Developing AI for local languages brings numerous hurdles, with the most glaring issue being a simple lack of data. While English boasts a wealth of linguistic data, Indian languages are severely underrepresented. Although Indian languages are spoken by 1.5 billion people, they only constitute about 4% of language data available—compared to English’s 67% with its 450 million speakers. This disparity is a major bottleneck in training AI models.
Linguistic diversity adds another layer of complexity. India is home to numerous languages and dialects, each with its own syntax, grammar, and vocabulary. This diversity means that any attempt at a one-size-fits-all approach to AI language processing isn’t going to work. Additionally, the contextual nuances and cultural connotations embedded in local languages make data annotation a truly challenging task, requiring a deep understanding of the language’s intricacies.
The limited availability of pre-training data makes things even more difficult. This data is essential for training AI models, yet there is a major scarcity of such data for many local languages. This is partly due to the limited number of experts who can accurately annotate data in these languages, but also due to the time-consuming and labor-intensive nature of the process.
The annotation solution: SoftAge AI
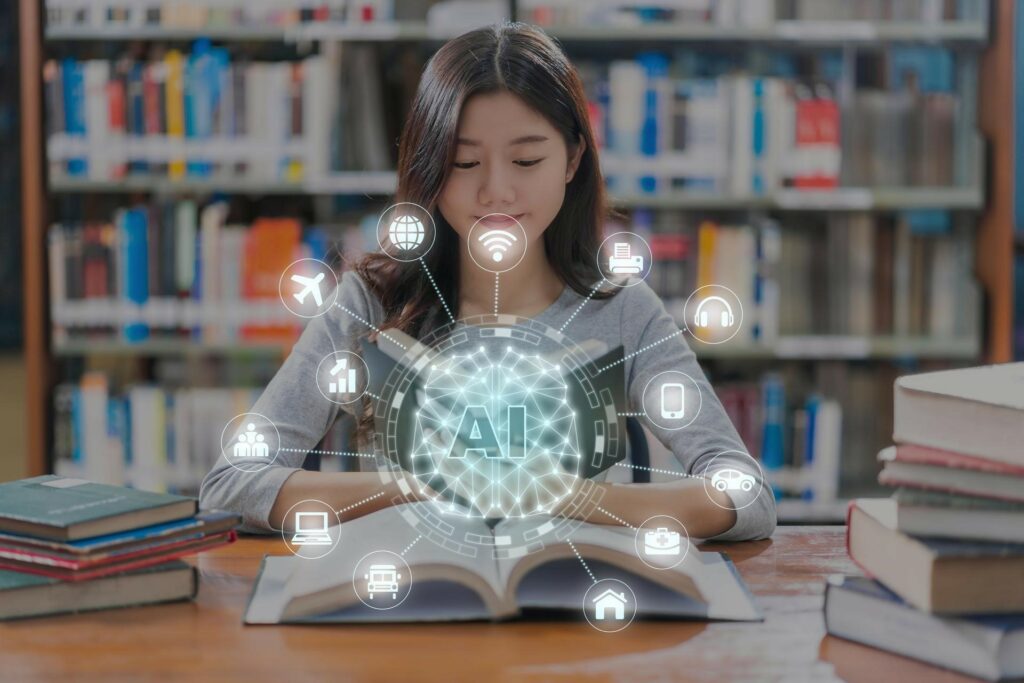
To bridge the annotation gap, platforms like SoftAge AI are looking to efficiently collect linguistic data from native speakers, allowing AI models to be trained to understand and respond accurately. It’s an involved process that involves several variables.
Community engagement
Getting local communities involved will help gather diverse and authentic language data, with social media campaigns, local events, and partnerships with educational institutions central to the process. Encouraging community participation will not only aid in data collection but nurture a sense of ownership and inclusivity among the speakers of local languages. Tapping into the knowledge and experiences of native speakers, SoftAge AI can gather valuable spoken and written data, vastly improving the quality and accuracy of AI models.
Government initiatives
Governments possess vast amounts of data in sectors like education, healthcare, and administration, which can be used for AI training. Partnering with governmental organizations, SoftAge AI can access these resources and integrate them into its data annotation processes. These collaborations can also help align AI initiatives with national policies and objectives for a more coordinated and effective approach. With governments providing the necessary funding and infrastructure to scale up data annotation efforts, the process can be dramatically accelerated.
Public domain and open data
The public domain is a veritable treasure trove of linguistic data. Texts, audio, and video from sources like old books, government publications, and free-to-air media provide a rich repository of information. Using these resources can help build a substantial dataset for AI training. SoftAge AI can systematically extract and annotate this data, so it’s structured and usable for AI models. This approach saves time and resources while tapping into a wealth of historical and cultural knowledge.
Web scraping
Extracting data from websites, forums, social media, and blogs in Indian languages is another way that SoftAge AI can gather a diverse array of language samples. That said, it’s a process that must be conducted ethically and legally, respecting privacy and data protection regulations. If scraping is performed conscientiously, it can provide real-time data that captures the evolving nature of a language and keeps AI models up-to-date.
SoftAge AI: A virtual Rosetta Stone
The journey towards achieving seamless multilingual AI in India is ongoing, but the progress made so far shows great promise. Through SoftAge AI’s coordination of expanded data annotation efforts and strengthened partnerships, the vision of an AI that seamlessly communicates in all local languages is becoming tangible.
The benefits this will bring to countries like India are far-reaching, from improving digital inclusivity to preserving linguistic heritage and allowing more effective communication across diverse populations. English may have served as an international standard for years (the fact that you’re reading this in English is no coincidence), however, given the potential of AI to completely transcend language barriers, it’s perhaps time that we left English to the English and gave AI the power to communicate with everyone on an accurate, one-to-one basis.
About SoftAge AI
SoftAge AI has been a leader in data management since its inception. Between 2008 and 2014, the company grew from 20 to 14,000 employees, processing over 2.5 billion data for more than 150 clients. In 2022, SoftAge expanded into AI data services, using its expertise to offer advanced solutions in language and agent models.