Large Language Models (LLMs) like GPT and BERT are changing the way we interact with technology on a near-daily basis. While the remarkable fluency with which these models can understand and generate human language may be impressive, it hides a fundamental problem. Unfortunately, GPT and its buddies don’t actually think for themselves yet and are instead trained on vast datasets that often reflect inherent biases in society. If GPT had been around in the 14th century, it would have confidently told you that migraines can be cured by drilling a hole in the skull to release the ‘evil spirits’ that are causing the pain.
In short, these models just regurgitate the thinking of the time without a thought in their digital minds about whether the information they’re providing is correct or not. In this article, we’ll explore how bias permeates these AI platforms and what can be done to address it.
The origins of bias in Large Language Models
At the heart of every LLM is data—massive amounts of it collected from sources like Wikipedia, news articles, books, and other publicly available content. These datasets shape how the model understands and processes language. However, language data isn’t neutral. It reflects the perspectives, priorities, and biases of its creators, who may belong to specific demographics, cultures, or political groups. When LLMs learn from these sources, they inevitably inherit the same biases.
For instance, a question like “What is a healthy breakfast?” might yield responses centered around Western food culture, such as oatmeal. While this is certainly popular in certain regions, it may not be culturally relevant to users from different parts of the world.
Social biases and their real-world impact
Bias in LLMs isn’t just a technical issue, it has real-world consequences. Social biases—ranging from gender and race to age and ethnicity—are often embedded in the data that LLMs learn from. Of these, gender bias is perhaps the most visible example. Many LLMs, when asked to generate text or perform tasks like translation, associate certain professions with a specific gender, reinforcing stereotypes. For instance, models often pair “doctor” with “he” and “nurse” with “she,” reflecting traditional but outdated gender roles.
Age bias also creeps into LLMs, with words like “young” often viewed more favorably than “old,” perpetuating negative perceptions of aging. With these biases influencing everything from job opportunities to social interactions, they can easily exacerbate existing inequalities.
Another challenge arises in the form of language and cultural bias. Most LLMs are predominantly trained in high-resource languages like English, leaving low-resource languages underrepresented. As a result, these models perform significantly better in well-represented languages, further marginalizing people who speak less widely used languages.
The challenge of reducing bias
Addressing bias in LLMs is no quick fix. Although there have been efforts to modify the architecture and training procedures of these models, the problem often lies deep in the data itself. Data is riddled with biases—both historical and contemporary—and models trained on this data will inevitably reflect these biases unless significant interventions are made.
One approach to reducing bias is domain adaptation, which involves fine-tuning LLMs on smaller, more balanced datasets that better represent diverse perspectives. However, this approach is resource-intensive and often only scratches the surface.
Unfortunately, bias is a deep-rooted human phenomenon, and addressing it requires a multifaceted approach that includes fields like psychology, linguistics, sociology, and economics. Understanding how these forces interact with language models may provide a clearer picture of the problem and lead to more effective solutions.
The way forward
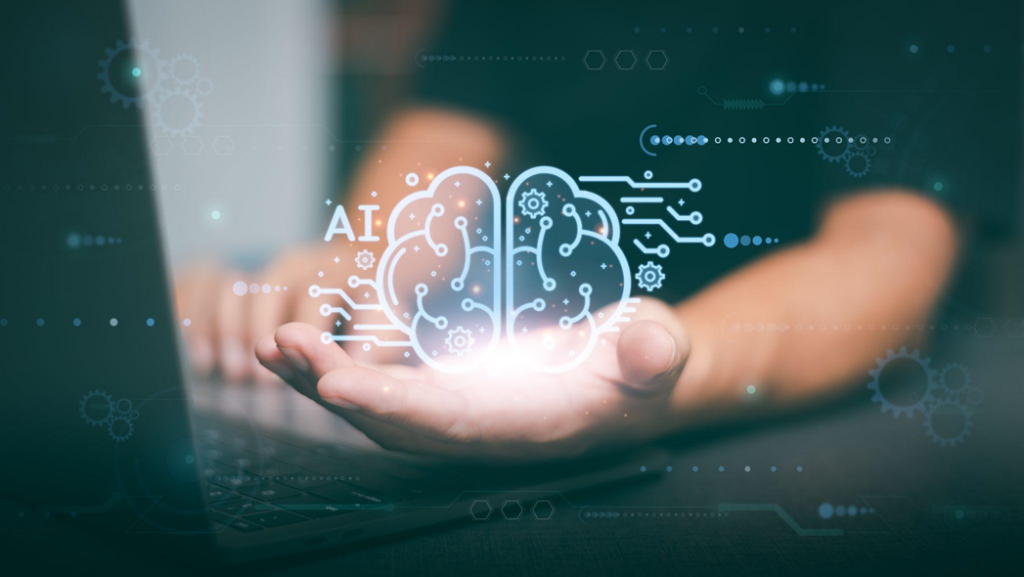
In order to remove the bias that permeates LLMs, we need to prioritize cultural and linguistic diversity. This involves expanding data sources to include a wider array of languages and cultural contexts while being mindful of the demographic diversity of content creators (the majority of Wikipedia editors, for example, are male and Western).
Broadening the scope of training datasets will allow LLMs to better represent the rich tapestry of global identities and experiences. Future research should focus on understanding the intent behind language rather than just its superficial structure. With context essential in assessing bias, models that grasp this nuance are less likely to perpetuate harmful stereotypes.
Ultimately, AI is a reflection of ourselves—at least for the moment. One day it may well evolve into a truly independent-minded system. In the meantime, the GPTs of the world will only ever be as ethical and inclusive as we are. If we teach AI well now, should it finally mature and decide to move out of its ‘parents’ house’, we can be hopeful it will be as responsible and thoughtful as it should be.
About SoftAge AI
SoftAge AI specializes in data management and AI-driven solutions, with a strong focus on linguistic diversity. Leveraging its extensive experience and innovative approaches, SoftAge AI is advancing digital transformation in India while preserving its rich cultural and linguistic heritage.